RSNA STR Chest CT Pulmonary Embolism Detection Dataset
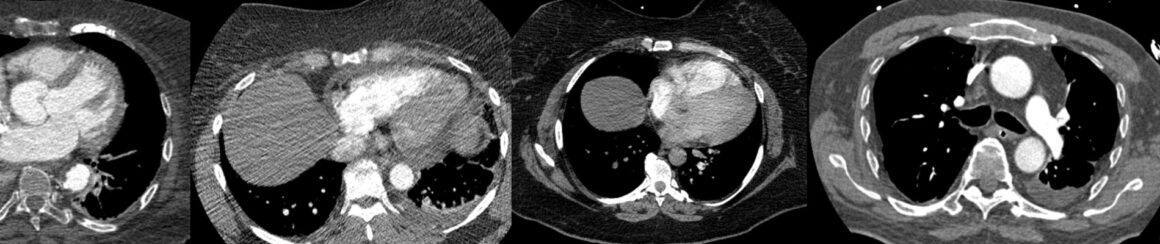
This dataset comprises 1,937,450 DICOM images (totaling 980.24 GB) drawn from 7,279 training studies, 650 public test studies, and 1,517 private test studies in the RSNA Pulmonary Embolism Detection Challenge. Each image is uniquely identified by a combination of StudyInstanceUID, SeriesInstanceUID, and SOPInstanceUID. Competitors predict various image-level and study-level labels related to pulmonary embolisms, including pe_present_on_image, negative_exam_for_pe, and several other attributes (e.g., rv_lv_ratio_gte_1, rightsided_pe, central_pe).
While the training data (train folder and train.csv) will not be accessible in inference-only kernels, participants must generate and incorporate models externally, then format predictions according to sample_submission.csv and test.csv. The labels follow a strict hierarchy, with certain labels being mutually exclusive and others purely informational (e.g., qa_motion, qa_contrast). All DICOM images include metadata that can assist in model development, and use of the data is subject to the RSNA-STR PE CT (RSPECT) dataset citation and non-commercial terms. 1Anouk Stein, MD, Carol Wu, Chris Carr, Errol Colak, George Shih, JeffRudie, John Mongan, Julia Elliott, Luciano Prevedello, Marc Kohli, MD, Phil Culliton, and Robyn Ball. RSNA STR Pulmonary Embolism Detection. https://kaggle.com/competitions/rsna-str-pulmonary-embolism-detection, 2020. Kaggle.
RSNA Brain CT Intracranial Hemorrhage Detection Dataset
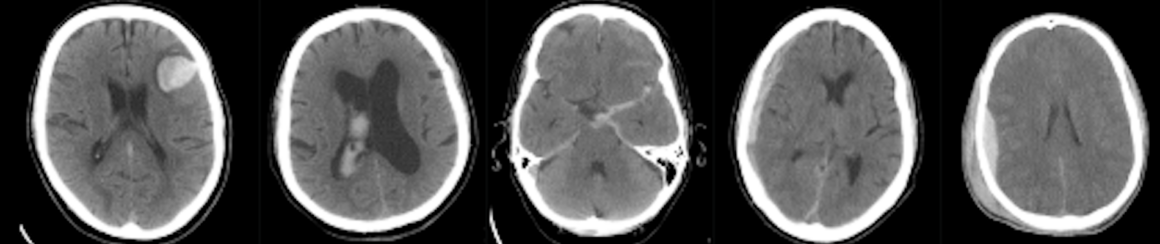
This dataset comprises 874,037 DICOM images (totaling 458.97 GB), each labeled to indicate the presence of five sub-types of hemorrhage (epidural, intraparenchymal, intraventricular, subarachnoid, and subdural) plus an overall “any” label. Every image is associated with six rows in the training file (stage_2_train.csv), each row containing a probability (Label) for one hemorrhage sub-type or for “any” if one or more hemorrhages are present.
Competitors must predict these probabilities for new images in the test set, which is provided in stage_2_test.zip. DICOM metadata (e.g., PatientID, StudyInstanceUID) is included with each image, and a sample submission (stage_2_sample_submission.csv) illustrates how to format predictions for the test set. 2Anouk Stein, MD, Carol Wu, Chris Carr, George Shih, Jayashree Kalpathy-Cramer, Julia Elliott, kalpathy, Luciano Prevedello, Marc Kohli, MD, Matt Lungren, Phil Culliton, Robyn Ball, and Safwan Halabi MD. RSNA Intracranial Hemorrhage Detection. https://kaggle.com/competitions/rsna-intracranial-hemorrhage-detection, 2019. Kaggle.
RSNA Chest X-Ray Pneumonia Detection Dataset
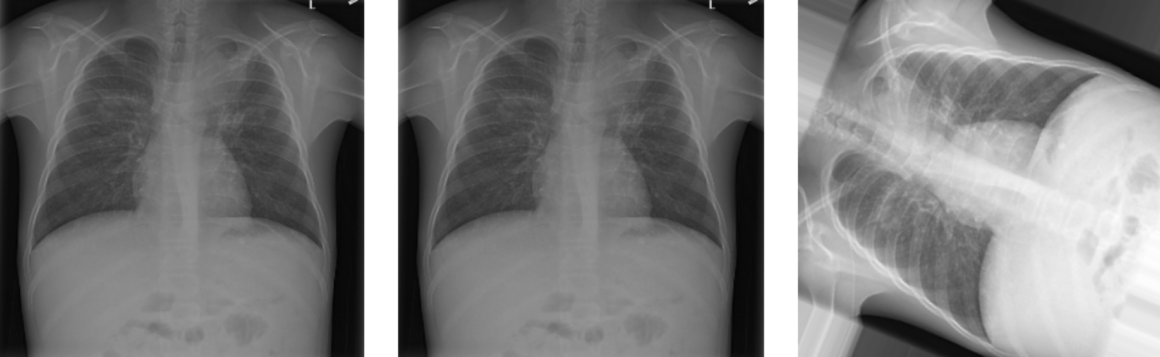
This dataset provides a collection of DICOM images alongside corresponding labels that indicate whether each image contains evidence of pneumonia. For each image, one or more bounding boxes may be present to localize suspected pneumonia, with coordinates (x-min, y-min, width, height) and an associated confidence score.
Multiple files are included: the train and test image sets (stage_2_train_images.zip and stage_2_test_images.zip), a main training label file (stage_2_train_labels.csv) that specifies each patientId, bounding box coordinates, and a binary pneumonia Target, a sample submission file to illustrate prediction formatting, and a detailed class information file that breaks down positive and negative classes more granularly. 3Anouk Stein, MD, Carol Wu, Chris Carr, George Shih, Jamie Dulkowski, kalpathy, Leon Chen, Luciano Prevedello, Marc Kohli, MD, Mark McDonald, Peter, Phil Culliton, Safwan Halabi MD, and Tian Xia. RSNA Pneumonia Detection Challenge. https://kaggle.com/competitions/rsna-pneumonia-detection-challenge, 2018. Kaggle.
Breast Ultrasound Images Dataset
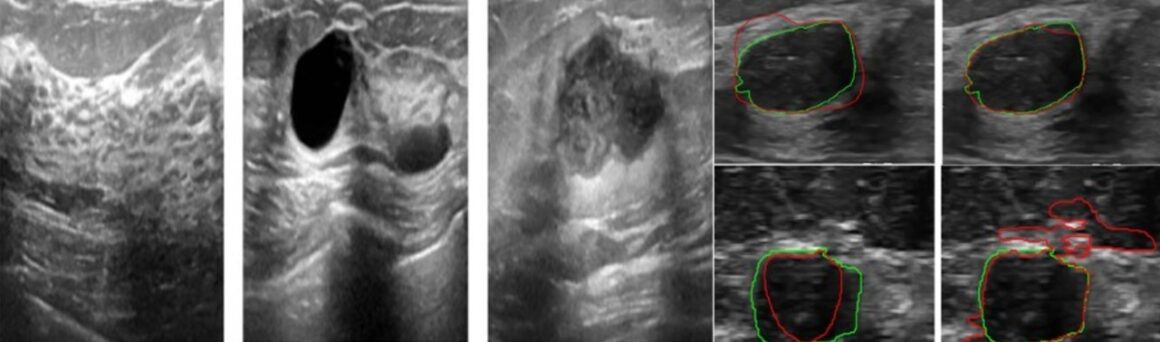
The data collected at baseline includes breast ultrasound images of women aged 25 to 75 years old. This data was gathered in 2018 and comprises 600 female patients. The dataset consists of 780 images, each with an average size of 500*500 pixels. The images are stored in PNG format. The ground truth images are presented alongside the original images. The images are categorized into three classes: normal, benign, and malignant. 4Al-Dhabyani W, Gomaa M, Khaled H, Fahmy A. Dataset of breast ultrasound images. 4Al-Dhabyani W, Gomaa M, Khaled H, Fahmy A. Dataset of breast ultrasound images. Data in Brief. 2020 Feb;28:104863. DOI: 10.1016/j.dib.2019.104863.
References
- 1Anouk Stein, MD, Carol Wu, Chris Carr, Errol Colak, George Shih, JeffRudie, John Mongan, Julia Elliott, Luciano Prevedello, Marc Kohli, MD, Phil Culliton, and Robyn Ball. RSNA STR Pulmonary Embolism Detection. https://kaggle.com/competitions/rsna-str-pulmonary-embolism-detection, 2020. Kaggle.
- 2Anouk Stein, MD, Carol Wu, Chris Carr, George Shih, Jayashree Kalpathy-Cramer, Julia Elliott, kalpathy, Luciano Prevedello, Marc Kohli, MD, Matt Lungren, Phil Culliton, Robyn Ball, and Safwan Halabi MD. RSNA Intracranial Hemorrhage Detection. https://kaggle.com/competitions/rsna-intracranial-hemorrhage-detection, 2019. Kaggle.
- 3Anouk Stein, MD, Carol Wu, Chris Carr, George Shih, Jamie Dulkowski, kalpathy, Leon Chen, Luciano Prevedello, Marc Kohli, MD, Mark McDonald, Peter, Phil Culliton, Safwan Halabi MD, and Tian Xia. RSNA Pneumonia Detection Challenge. https://kaggle.com/competitions/rsna-pneumonia-detection-challenge, 2018. Kaggle.
- 4Al-Dhabyani W, Gomaa M, Khaled H, Fahmy A. Dataset of breast ultrasound images. 4Al-Dhabyani W, Gomaa M, Khaled H, Fahmy A. Dataset of breast ultrasound images. Data in Brief. 2020 Feb;28:104863. DOI: 10.1016/j.dib.2019.104863.